Cluster Sampling: Definition, Steps, Types & Examples
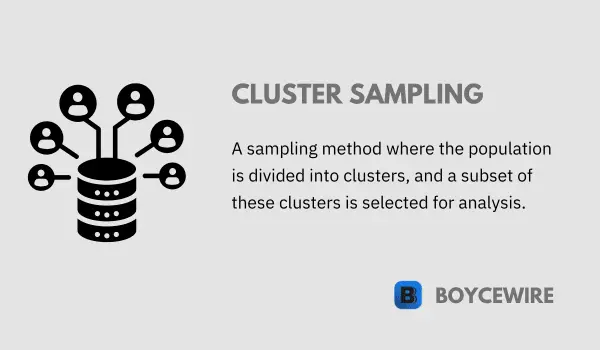
What is Cluster Sampling?
Sampling is a critical process in statistics, research, and data analysis, allowing investigators to draw conclusions about a population without studying every individual within it. One of the common methods used in various fields – from market research to public health studies – is cluster sampling.
Cluster sampling is a method of probability sampling where a researcher divides the population of interest into separate groups, known as clusters. A subset of these clusters is then randomly selected for further study. Each cluster should ideally be a microcosm of the population, containing a variety of respondents that represent different subgroups of the population.
This technique is especially useful in large-scale studies where the population is widespread geographically and conducting a simple random sample would be impractical or cost-prohibitive. By focusing on specific clusters, researchers can save time and resources while still collecting valuable, representative data.
Key Points
- Cluster sampling is a sampling technique where the population is divided into clusters or groups.
- Instead of selecting individual elements, entire clusters are randomly chosen for data collection.
- It is often used when it is more practical or cost-effective to sample groups of individuals.
Understanding Cluster Sampling
Cluster sampling involves breaking down a population into separate groups, or clusters, based on certain criteria. These clusters are then randomly selected, and all individuals within the chosen clusters are included in the study.
In one-stage cluster sampling, a random sample of clusters is selected, and all individuals within those clusters are included in the study. In two-stage cluster sampling, after selecting the clusters, a random sample of individuals within those clusters is drawn.
The key principle is that each cluster should be a representative microcosm of the population. This ensures that the results can be generalized to the whole population.
It is important to note that it differs from stratified sampling, where random samples are drawn from each subgroup (or stratum) of the population. In cluster sampling, entire clusters are studied.
Steps in Cluster Sampling
Cluster sampling may seem complicated, but it can be broken down into a series of simple steps:
- Define the Population The first step in any sampling process is to clearly define the population you’re studying. The population is the total group that you want to draw conclusions about.
- Identify the Clusters Once you have defined your population, the next step is to identify potential clusters. Clusters are groups or categories that exist within the population. They might be defined geographically, demographically, or based on some other criteria that’s relevant to your study.
- Select the Clusters After identifying the clusters, the next step is to select which clusters you’ll actually study. This is typically done randomly to avoid bias. You may choose to use either a one-stage or a two-stage sampling process. In one-stage cluster sampling, you would include all members of the selected clusters in your study. In two-stage cluster sampling, you would further randomly select specific individuals within the chosen clusters to include in your study.
- Collect the Data Once you’ve selected your clusters, the next step is to collect your data. This could involve surveys, interviews, observations, or any other data collection method.
- Analyze the Data After data collection, you’ll analyze your data to draw conclusions about your population. When using cluster sampling, it’s essential to take into account the cluster design during the analysis phase, using statistical methods that recognize the clustered nature of the data.
- Draw Conclusions The final step is to draw conclusions based on your analysis. Because you’ve used a form of probability sampling (assuming you’ve selected your clusters randomly), you should be able to generalize your results to the population as a whole.
Types of Cluster Sampling
Cluster sampling can be mainly categorized into two types:
- One-stage Sampling In this type of cluster sampling, the researcher selects clusters for the sample and includes all the elements within the selected clusters for the study. For instance, if a researcher is studying the performance of students in a school district and selects five schools as clusters, under one-stage cluster sampling, they would include all students from these five schools in their study.
- Two-stage Sampling This method involves a second stage of random sampling within the selected clusters. Using the same example of a researcher studying student performance, in two-stage cluster sampling, after selecting five schools, they would then randomly select a certain number of students from each school to include in the study.
Both methods have their advantages and can be more suitable in certain scenarios. One-stage sampling is simpler and less costly but might lead to less precise results. On the other hand, two-stage sampling can provide more precise results but is more complex and could be more expensive due to the added layer of random selection.
Advantages of Cluster Sampling
Cluster sampling offers several advantages that can make it an attractive choice for researchers:
- Cost-effective This method can be more cost-effective than other forms of sampling because it minimizes travel and administrative costs. This is especially beneficial when the population is spread over a large geographic area.
- Efficiency Cluster sampling can be more efficient than other methods. Once clusters are chosen, researchers can collect data from a large number of respondents in one area, reducing the time and resources needed to reach individual subjects scattered across different locations.
- Convenience and Practicality This type of sampling can be a more convenient and practical option when it is difficult to compile an exhaustive list of all members of the population. For instance, if you are surveying the residents of a city, it would be far easier to identify all the neighborhoods (clusters) and then sample within them, rather than trying to compile a list of every resident.
- Representativeness If clusters are chosen well, they can maintain the diversity of the population, resulting in a sample that is highly representative of the population as a whole. This is particularly the case with two-stage sampling, where the second stage allows for random sampling within each cluster.
Disadvantages of Cluster Sampling
While cluster sampling has many advantages, it is also important to consider the limitations that come with this method:
- Bias and Error Cluster sampling can introduce bias and increase sampling error. This happens because members within the same cluster may have similar characteristics, leading to less variability and diversity compared to the entire population. As a result, the sample may not be as representative as it could be with other sampling techniques.
- Difficulty in Choosing Clusters It can be difficult to define and choose appropriate clusters, particularly if there’s a lack of preliminary information about the population. Improper selection of clusters may lead to inadequate representation of the population and skewed results.
- Large Sample Size Required Compared to other methods like simple random sampling, cluster sampling typically requires a larger sample size to achieve the same level of precision. This is due to the intra-cluster correlation.
- Analysis Complexity Analyzing data collected through a sampling method that incorporates clustering can present additional complexities, as it requires accounting for the clustered structure of the sample. Traditional statistical techniques may need to be adapted or substituted with more advanced methods to ensure the validity of the results.
Despite these disadvantages, this type of sampling can still be a useful tool in the hands of a skilled researcher who understands its limitations. The technique’s efficiency and cost-effectiveness can often outweigh the potential drawbacks, especially in large-scale field studies.
Applications of Cluster Sampling
Cluster sampling is widely used across various disciplines due to its efficiency and cost-effectiveness. Here are a few examples of its applications:
- Public Health In the field of public health, researchers often use such sampling to conduct surveys and health assessments on a large scale. For example, if a health agency wants to assess vaccination rates in a large city, they could divide the city into different neighborhoods (clusters), randomly select a few neighborhoods, and then sample individuals within those chosen clusters.
- Market Research Businesses may use cluster sampling to gather customer feedback or understand purchasing behaviors. For example, a company might divide its market into geographic clusters (such as cities or regions), then select a subset of these clusters for detailed research.
- Environmental Studies In environmental science, this type of sampling can be useful when collecting data across large geographical areas. For instance, to study deforestation, researchers could divide a forest into clusters (e.g., square kilometers) and then randomly select certain clusters for detailed analysis.
- Education In educational research, sampling using clusters is often employed when data from schools or universities are collected. The institution itself serves as the cluster. For instance, if a study aims to measure the impact of a new teaching method, researchers could randomly select a few schools out of a school district and then collect data from all teachers in those schools.
Examples of Cluster Sampling
- Census Surveys When conducting a national survey, it is often impractical to survey every individual due to resource constraints. In this case, the country can be divided into different clusters (like states or provinces), a few clusters are randomly selected, and every household within these clusters is surveyed.
- Educational Surveys Suppose an educational board wants to assess the effectiveness of a new teaching method. The board can group schools into different clusters based on their location (urban or rural), randomly pick a few from each group, and then survey all the teachers in the selected schools.
- Environmental Studies Consider a scenario where a researcher wants to study the vegetation of a large forest. The forest can be divided into different clusters (based on geographical areas). Then, a few of these clusters can be randomly chosen for the study.
- Marketing Research A company wants to launch a new product and needs to know the potential consumer response. It can divide its target market into clusters (based on geographic location or demographic characteristics), randomly select a few clusters, and conduct the research within those clusters.
- Public Health Research For example, if the World Health Organization wants to estimate the prevalence of a disease in a country, it might divide the country into clusters (regions or districts), randomly select a few clusters, and then survey all households within the selected clusters.
FAQs
Cluster sampling is a sampling method in which the population is divided into clusters or groups, and a subset of these clusters is selected for data collection.
Unlike simple random sampling where individual elements are selected randomly, cluster sampling involves selecting entire clusters as the sampling units.
Cluster sampling is often used when it is more practical or cost-effective to sample groups of individuals rather than selecting each individual separately. It is also useful when the population is naturally grouped into clusters.
Cluster sampling can be more efficient in terms of time and cost, as it allows for sampling large populations by focusing on selected clusters. It can also provide a good representation of diverse subgroups within the population.
One limitation is the potential for increased sampling error due to the similarity of individuals within clusters. Additionally, if the clusters are not representative of the overall population, the sample results may be biased.
About Paul
Paul Boyce is an economics editor with over 10 years experience in the industry. Currently working as a consultant within the financial services sector, Paul is the CEO and chief editor of BoyceWire. He has written publications for FEE, the Mises Institute, and many others.
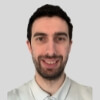
Further Reading
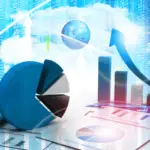
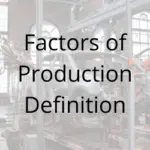
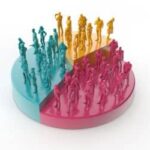