Chi Square Test: Definition & How to Calculate
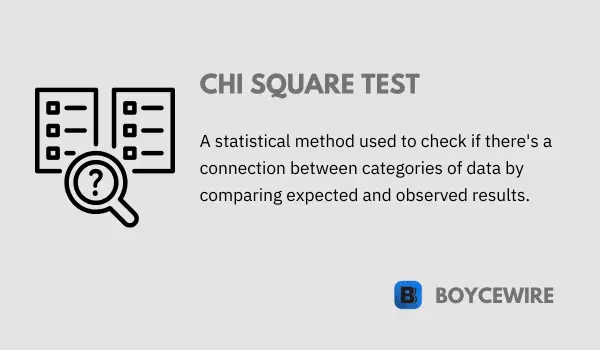
What is a Chi Square Test?
The field of statistics offers us various tools to make sense of data and draw meaningful conclusions from it. One such powerful tool is the Chi-Square Test. This non-parametric test is widely used in research and data analysis to test hypotheses about the relationship between categorical variables, compare observed data with expected data, or test the homogeneity or independence of two categorical variables.
The Chi-Square Test is named after the Greek letter “Chi” (χ) due to its association with the Chi-Square distribution, which is a fundamental concept in this test. The beauty of this test lies in its simplicity and versatility, making it a popular choice across numerous disciplines, from biology to marketing research, psychology to social sciences, and many more.
Key Points
- The chi-square test is a statistical test used to analyze categorical data and determine if there is a significant association between two or more variables.
- It compares observed frequencies of data to expected frequencies to assess whether any deviation from expected values is statistically significant.
- The test is applicable when data are organized into categories or groups, and the variables of interest are independent of each other.
Understanding the Chi-Square Test
The Chi-Square Test is a statistical method used to determine if there is a significant association between two categorical variables in a sample. It falls under the category of non-parametric tests, meaning it doesn’t make assumptions about the population parameters and the distribution of the variables.
In its simplest form, the Chi-Square Test compares the observed data in a study with the data we would expect to obtain according to a specific hypothesis. It’s essentially a measure of how much observed frequencies deviate from expected frequencies.
There are three types of Chi-Square Tests, each serving a different purpose:
- Chi-Square Goodness of Fit Test
- Chi-Square Test of Independence
- Chi-Square Test of Homogeneity
In a Chi-Square Test, we first formulate a null hypothesis (H0) which usually states that there is no relationship between the variables (independence) or that the data fits the distribution (goodness of fit). The alternative hypothesis (H1) states the contrary, i.e., there is a relationship between the variables or the data does not fit the distribution. After conducting the test, if the resulting p-value is less than our significance level (commonly 0.05), we reject the null hypothesis.
In the upcoming sections, we’ll delve into how the Chi-Square statistic is calculated, its distribution, and how to interpret the results of a Chi-Square Test.
The Chi-Square Distribution
Understanding the Chi-Square distribution is essential to comprehend the Chi-Square Test. It is a particular case of the Gamma distribution and has its roots in the method of calculating the Chi-Square Test’s statistic.
The Chi-Square distribution is a family of probability distributions that is defined by one parameter, known as the degrees of freedom. The degrees of freedom for a Chi-Square distribution is an important concept and typically equals the number of categories in the data minus one. The shape of the Chi-Square distribution changes based on the degrees of freedom.
A few key characteristics of the Chi-Square distribution are:
- Shape: The Chi-Square distribution is skewed to the right. This skewness decreases as the degrees of freedom increase. With enough degrees of freedom, the distribution tends towards a normal distribution.
- Non-Negativity: The Chi-Square distribution is only defined for positive values. This makes sense because in the context of a Chi-Square Test, we’re summing the squares of quantities, which can’t be negative.
- Degrees of Freedom: This is a crucial parameter that determines the shape of the Chi-Square distribution. Degrees of freedom are often equivalent to the number of categories or groups in the data minus one.
- Mean and Variance: The mean of a Chi-Square distribution is equal to its degrees of freedom, and the variance is twice the degrees of freedom.
In a Chi-Square Test, we calculate a Chi-Square statistic and compare it to a Chi-Square distribution with the appropriate degrees of freedom. This comparison allows us to derive a p-value and determine the statistical significance of the test result. If the calculated Chi-Square statistic is large enough (based on the p-value and our predetermined significance level), we conclude that the observed data significantly deviates from what was expected under the null hypothesis.
Calculating the Chi-Square Statistic
The process of calculating the Chi-Square statistic involves several steps, mainly based on the observed and expected frequencies of the data.
First, let’s understand these two types of frequencies:
- Observed Frequencies (O): These are the actual frequencies observed in the data. For example, in a study investigating the relationship between gender and product preference, the observed frequencies would be the actual number of men and women who preferred each product.
- Expected Frequencies (E): These are the frequencies we would expect if there were no relationship between the variables – in other words, if the null hypothesis is true. In the same example, the expected frequencies would be the number of men and women who would prefer each product if product preference was independent of gender.
Once you have the observed and expected frequencies, you can calculate the Chi-Square statistic (χ²) using the following formula:
χ² = Σ [ (O-E)² / E ]
Here’s a step-by-step guide to calculate the Chi-Square statistic:
- Compute the Expected Frequencies: For each category, calculate the expected frequency. If you’re testing for independence, the expected frequency for each cell in a contingency table is (row total * column total) / grand total.
- Calculate the Chi-Square Statistic: Subtract the expected frequency from the observed frequency for each category, square the result, then divide by the expected frequency. Do this for all categories.
- Sum the Values: Add up all the values calculated in step 2. This sum is your Chi-Square statistic.
Remember that the Chi-Square Test is always a one-tailed test. The distribution is not symmetric, and we are only interested in whether the observed test statistic is in the tail of the distribution compared to the critical value.
It’s important to note that each of the expected frequencies should be 5 or more for the Chi-Square approximation to be valid. If the expected frequencies are too small, the Chi-Square Test may not be appropriate.
Interpreting Chi-Square Test Results
After calculating the Chi-Square statistic, the next step is to interpret the results to determine whether the observed data are statistically significantly different from the expected data.
The interpretation of Chi-Square Test results revolves around the p-value. A p-value is a probability that measures the evidence against the null hypothesis. A smaller p-value provides stronger evidence against the null hypothesis.
Here is how to interpret the results:
- Calculate the P-value: Using the Chi-Square distribution table or a statistical software, determine the p-value associated with your calculated Chi-Square statistic and the appropriate degrees of freedom. The degrees of freedom for a Chi-Square test is usually calculated as (number of rows – 1) * (number of columns – 1) in your contingency table.
- Compare P-value to Significance Level: The common threshold (alpha level) used in many fields is 0.05 (5%). If your p-value is less than 0.05, you reject the null hypothesis. In the context of a Chi-Square Test, this would mean there is a statistically significant difference or relationship.
- If P-value < 0.05: There is less than a 5% chance that the differences or relationship you observed in your data occurred by chance alone. You reject the null hypothesis and conclude that there is a significant difference or association.
- If P-value ≥ 0.05: There is a greater than 5% chance that the differences or relationship you observed in your data occurred by chance alone. You do not reject the null hypothesis and conclude that there is not a significant difference or association.
Remember, the Chi-Square Test only tells us if there is a relationship between the variables, not how strong or weak the relationship is. For measuring the strength and direction of the association, other statistical measures like Cramer’s V or Phi coefficient might be more suitable.
It’s also important to remember that “statistically significant” does not necessarily mean “practically significant.” The distinction depends on the subject matter and research context. It is possible to have a result that is statistically significant but not practically meaningful, especially with large sample sizes.
Assumptions of the Chi-Square Test
Like all statistical tests, the Chi-Square Test also operates under certain assumptions. When these assumptions are met, the test results are valid. Here are the main assumptions for the Chi-Square Test:
- Independence: The observations should be independent of each other. This means that the presence or absence of a characteristic in one participant or group should not influence the presence or absence of it in another. For example, one person choosing a particular product should not influence another person’s choice.
- Randomness: The data should come from a random sample or a randomized experiment. This ensures that the sample is representative of the population, and the results of the test can be generalized to the population.
- Categorical Data: The Chi-Square Test is used for categorical or nominal data. This includes variables like color, brand, preference, yes/no responses, etc. It cannot be used for numerical or ordinal data.
- Sufficient Sample Size: In order for the Chi-Square distribution to approximate the distribution of the test statistic well, the sample size must be sufficiently large. A common rule of thumb is that each cell in the contingency table should have an expected count of at least 5.
- Fixed Marginals: In the Chi-Square Test for independence, it is assumed that the marginal totals (i.e., the row and column totals in the contingency table) are fixed in advance. This may not always be the case in some observational studies.
Violating these assumptions can lead to misleading results. For instance, if the sample size is too small or the data does not meet the expected frequency assumption, the test might be underpowered or yield inaccurate p-values. If assumptions are violated, other types of statistical tests might be more appropriate.
Limitations of the Chi-Square Test
- The Chi-Square Test can only be used on categorical (nominal) or ordinal data. It cannot be used to analyze continuous or numerical data.
- The Chi-Square Test assumes that the categories or groups are mutually exclusive, meaning an observation can belong to one and only one category or group. If categories overlap, the test may not be appropriate.
- While it’s considered a non-parametric test and doesn’t require the assumption of normality, the Chi-Square Test still relies on the assumption that your expected frequencies are reasonably large. The rule of thumb is that all cells in a contingency table should have expected frequencies of at least 5. If you have smaller sample sizes, the test can still be conducted, but it may not be as reliable.
- The Chi-Square Test only assesses simple hypotheses such as independence or homogeneity. It doesn’t provide information about complex relationships or interactions between variables. Also, it does not give any insight into the direction or magnitude of associations.
- The Chi-Square Test is based on the comparison of observed to expected frequencies, which assumes that you know what to expect under the null hypothesis. In some cases, specifying these expected frequencies can be challenging.
- While the Chi-Square Test can tell you if there is a significant relationship between two categorical variables, it does not provide information about the strength or the direction of the relationship.
While these limitations should be taken into account, the Chi-Square Test remains a powerful tool for hypothesis testing with categorical data. Understanding its limitations helps ensure that it’s the appropriate test for your data and that your conclusions are valid.
FAQs
The chi-square test is used to determine if there is a significant association between categorical variables and to analyze the goodness-of-fit of observed and expected frequencies.
The chi-square test is applicable when working with categorical data, such as counts or proportions in different categories or groups.
The null hypothesis in the chi-square test states that there is no association between the variables or that the observed frequencies match the expected frequencies.
The chi-square test involves calculating the chi-square statistic by comparing observed frequencies with expected frequencies based on the null hypothesis. This statistic is then compared to a critical value or p-value to determine statistical significance.
About Paul
Paul Boyce is an economics editor with over 10 years experience in the industry. Currently working as a consultant within the financial services sector, Paul is the CEO and chief editor of BoyceWire. He has written publications for FEE, the Mises Institute, and many others.
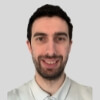
Further Reading
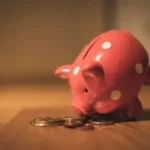
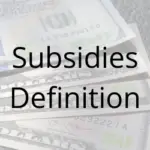
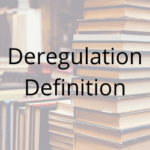