Empirical Rule: Definition, Formula & Examples
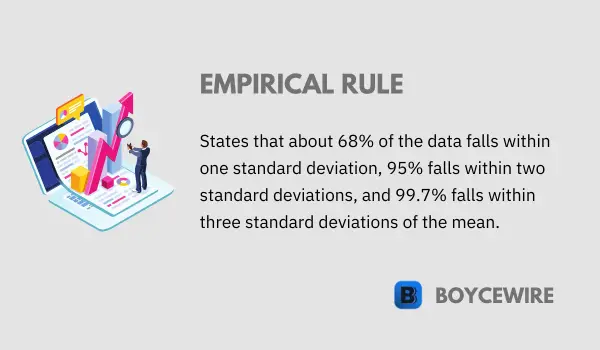
What is the Empirical Rule?
The Empirical Rule, also known as the 68-95-99.7 rule, provides a quick and relatively simple way to analyze and make sense of data sets, specifically those that follow a normal distribution. It enables economists to make estimates and predictions about data distribution and can aid significantly in the process of economic forecasting, risk assessment, and policy planning.
While the Empirical Rule is not without its limitations, its utility and application in economics cannot be overstated. This article will delve into the Empirical Rule, its relationship with normal distribution, its application in economics, and some real-world examples. We will also explore the limitations of the Empirical Rule and when alternative statistical tools may be more appropriate. Join us as we unfold the nuances of this fascinating statistical concept and its impact on the world of economics.
Key Points
- The empirical rule is a statistical guideline that applies to data that follows a normal distribution.
- It states that approximately 68% of the data falls within one standard deviation of the mean, 95% falls within two standard deviations, and 99.7% falls within three standard deviations.
- The empirical rule is based on the properties of a bell-shaped curve and is widely used to estimate the spread and distribution of data.
Understanding the Empirical Rule
The Empirical Rule, also known as the 68-95-99.7 rule, is a fundamental concept in statistics that applies to data sets following a normal distribution, or a bell curve. The rule derives its name from the observation that in a normal distribution:
- Approximately 68% of all data falls within one standard deviation from the mean.
- Approximately 95% of data falls within two standard deviations from the mean.
- Approximately 99.7% of data falls within three standard deviations from the mean.
But what does this mean? In essence, the Empirical Rule provides a rough estimate of where values in a data set are likely to be found, assuming the data is normally distributed. The ‘standard deviation’ is a measure of the dispersion or spread in a data set. So, the mean (or average) plus or minus a standard deviation will contain about 68% of all the data points. If you increase the range to two standard deviations, you’ll capture about 95% of the data, and increasing the range to three standard deviations will contain almost all the data points (99.7%).
The beauty of the Empirical Rule lies in its simplicity. It offers an intuitive and straightforward way to understand, predict, and interpret data patterns within a normal distribution, without the need for complex calculations or analyses. In the field of economics, this rule can be especially helpful when analyzing economic data, which often falls into normal (or near-normal) distributions.
Formula of the Empirical Rule
The Empirical Rule, in itself, does not have a specific mathematical formula. Instead, it provides a guideline for the distribution of data within a normal distribution. But you can apply the rule using the knowledge of the mean (average) and the standard deviation in your data set. Here’s how it works:
- One Standard Deviation: 68% of data falls within one standard deviation of the mean. Mathematically, this can be expressed as (µ – σ, µ + σ), where µ represents the mean and σ represents the standard deviation.
- Two Standard Deviations: About 95% of the data falls within two standard deviations of the mean. This can be expressed as (µ – 2σ, µ + 2σ).
- Three Standard Deviations: Approximately 99.7% of the data falls within three standard deviations of the mean. This can be represented as (µ – 3σ, µ + 3σ).
In all three cases, the values within the parentheses represent the range within which the specified percentage of data is expected to fall, assuming the data follows a normal distribution.
It’s important to remember that the Empirical Rule only applies to data sets that are normally distributed. If the data set is not normally distributed, the distribution of data will not necessarily follow these rules, and other statistical techniques may be more appropriate for data analysis.
Examples of the Empirical Rule in Economics
Let’s look at a few examples to illustrate how the Empirical Rule can be used in economics:
1. Income Distribution
Suppose we have a city where the average income is $50,000, and the standard deviation is $10,000. If we assume that income distribution follows a normal distribution, we can use the Empirical Rule to estimate that about 68% of people in the city earn between $40,000 and $60,000 (mean ± 1 standard deviation). Around 95% earn between $30,000 and $70,000 (mean ± 2 standard deviations), and virtually all (99.7%) earn between $20,000 and $80,000 (mean ± 3 standard deviations).
2. Commodity Prices
Let’s say we’ve collected monthly data over several years for the price of a certain commodity, like wheat. We find that the average price is $200 per ton with a standard deviation of $20. If we assume that the price data follows a normal distribution, we can use the Empirical Rule to estimate that in about 68% of the months, the price per ton will be between $180 and $220. In about 95% of the months, the price will be between $160 and $240, and in almost all (99.7%) of the months, the price will be between $140 and $260.
3. Stock Market Returns
Suppose an economist is studying the annual returns of a specific stock market index. She finds that the average return is 8% with a standard deviation of 2%. If the returns are normally distributed, she could use the Empirical Rule to predict that about 68% of the time, the annual return will be between 6% and 10%. Around 95% of the time, the return will be between 4% and 12%, and almost always (99.7% of the time), the return will be between 2% and 14%.Applying the Empirical Rule in Economics
Economists often use the Empirical Rule as a tool to understand and predict patterns in economic data. Given the diversity and volume of economic data available, the rule’s simplicity and quick application to normal distributions can provide valuable insights in a range of situations.
Here are several ways the Empirical Rule can be applied in economics:
- Risk Assessment: In finance and investment, the Empirical Rule can help assess the risk associated with a particular investment. By understanding how investment returns or stock prices are distributed, investors can make informed decisions about the level of risk they’re willing to take. The rule can help investors understand how much a stock’s price might deviate from the mean, giving them a sense of potential profit and loss.
- Economic Forecasting: Economists often use statistical models to forecast future economic conditions. By understanding the distribution of past economic data, such as GDP growth or unemployment rates, economists can make predictions about future trends. The Empirical Rule can provide a quick way to understand the distribution of data and can inform these forecasting models.
- Policy Planning: Policymakers can use the Empirical Rule to understand and predict outcomes of various social and economic phenomena, such as income distribution, unemployment rates, or educational attainment. This understanding can guide policy decisions and help predict the outcomes of different policy choices.
- Market Research: In market research, the Empirical Rule can help companies understand consumer behavior patterns. For instance, if a company gathers data on how much customers spend per visit and finds that the data is normally distributed, the Empirical Rule can help the company understand what range of spending to expect from most customers.
While the Empirical Rule is a powerful tool in economics, it’s important to remember that it’s a simplification. Real-world data can often deviate from the neat patterns predicted by the Empirical Rule, so economists often use it in combination with other statistical tools and methods to analyze economic data.
Limitations of the Empirical Rule
While the Empirical Rule is a useful tool in statistical analysis, it does have its limitations, particularly in its application to economics. Understanding these limitations is important for accurate interpretation and application of the rule.
- Normal Distribution Assumption: The Empirical Rule only applies to data that follows a normal distribution. In the real world, not all data, including economic and financial data, follows this pattern. For example, the distribution of wealth or income in a society often follows a skewed, not symmetric, distribution. In such cases, the Empirical Rule will not provide accurate predictions.
- Estimations, Not Exact Values: The rule provides estimates (68%, 95%, and 99.7%), not exact values. These are approximations and can slightly differ in real-world data sets.
- Uninformative for Outliers: The Empirical Rule is not informative for data beyond three standard deviations from the mean. If you have significant outliers in your data, the rule will not provide insights about these extreme values.
- Single Variable Analysis: The Empirical Rule is used for single variable analysis and does not account for interactions between multiple variables. In economics, variables are often interrelated, and analysis usually involves multiple variables.
- Ignorance of Central Tendency Measure: The Empirical Rule is completely dependent on the mean and standard deviation, but in skewed distributions, the median might be a better measure of central tendency.
Therefore, while the Empirical Rule is a valuable tool for understanding and predicting data trends, it should be used cautiously and in conjunction with other statistical tools for a more comprehensive analysis, especially when dealing with economic data.
FAQs
The empirical rule, also known as the 68-95-99.7 rule, is a statistical guideline that describes the approximate distribution of data in a normal distribution.
The empirical rule states that in a normal distribution, approximately 68% of the data falls within one standard deviation of the mean, 95% falls within two standard deviations, and 99.7% falls within three standard deviations.
The empirical rule provides a quick and useful way to estimate the spread and distribution of data in a normal distribution, helping to analyze and interpret data in various fields, including economics.
The empirical rule is often used in economic analysis to understand the distribution of economic variables, such as incomes, prices, and returns, and to make statistical inferences and predictions.
About Paul
Paul Boyce is an economics editor with over 10 years experience in the industry. Currently working as a consultant within the financial services sector, Paul is the CEO and chief editor of BoyceWire. He has written publications for FEE, the Mises Institute, and many others.
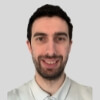
Further Reading
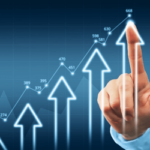
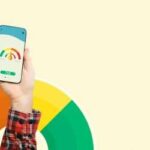
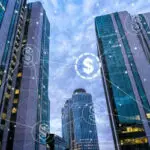