Effect Size: How to Calculate, Types & Examples
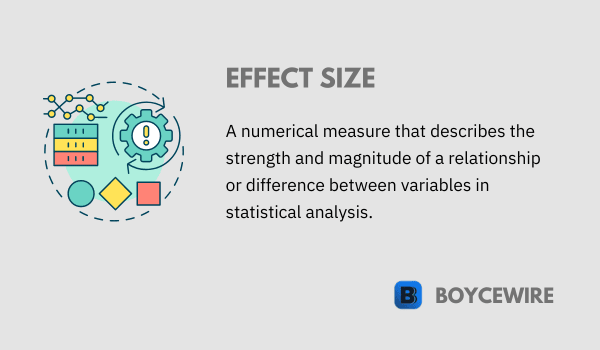
What is Effect Size?
Effect size is a key concept in statistical analysis, often used in conjunction with hypothesis testing. It provides a quantitative measure of the magnitude or size of an effect, going beyond simply determining whether the effect exists. In essence, while statistical significance can answer the question of ‘is there an effect?’ the effect size helps answer ‘how big is the effect?’
It is particularly important in research and experimentation as it offers a more comprehensive view of study results. Unlike p-values, which only suggest whether an effect exists, the effect size provides information about the practical significance of results, helping to determine if the findings are meaningful in real-world applications.
Key Points
- Effect size measures the magnitude or strength of the relationship between variables in a statistical analysis.
- It helps determine the practical significance of research findings and allows for comparisons across studies.
- Commonly used effect size measures include Cohen’s d, Pearson’s r, and odds ratios.
Understanding Effect Size
The effect size refers to a statistical concept that measures the strength or magnitude of a phenomenon or effect. In research studies, it is often used to quantify the difference between two groups or the relationship between two variables, providing a more complete picture of the results beyond what can be captured by a p-value alone.
The concept is rooted in the understanding that statistical significance is not synonymous with practical or clinical significance. A large sample size might lead to statistically significant results, even if the actual difference or relationship is small and perhaps not meaningful in a practical sense.
Conversely, in smaller studies, a large and potentially meaningful difference might not reach statistical significance. This is where the concept of effect size comes in. It provides a way to quantify the size or magnitude of an effect independently of the size of the study or the variability in the data.
The measure of effect size is particularly important for comparative studies. For instance, if two educational interventions are being tested, it’s not enough to know that one is effective while the other is not. Researchers, educators, and policymakers would likely be more interested in knowing how much more effective one is over the other, a question that can be answered by computing and interpreting the effect size.
Moreover, it can be used to determine the sample size required for a study, helping researchers in the planning stages of an experiment or observational study. Also, when the results of multiple studies are combined in a meta-analysis, effect sizes provide a common metric that allows for meaningful comparison and synthesis of findings across studies.
Types of Effect Size
- Cohen’s d Cohen’s d is used to measure the effect size in the difference between two means and is typically used in experiments. It represents the difference in means between two groups divided by the standard deviation from the data.
- Cohen’s f This is used in the context of ANOVA tests and is a measure of variance explained by the model, similar to the R2 in regression.
- Eta Squared (η²) Eta Squared is used in the context of ANOVA (Analysis of Variance) tests and represents the proportion of the total variance in a dependent variable that can be attributed to the variance in the grouping (i.e., independent) variables.
- Cohen’s h This effect size is used for proportions and rates. Cohen’s h is used to measure the difference between two proportions or rates in the same population.
- Odds Ratio (OR) The odds ratio is used in case-control studies for categorical data to provide a comparison of the odds of the occurrence of the event of interest (outcome) in one group to the odds of occurrence of the event in the other group.
- Relative Risk (RR) The relative risk is used in randomized control studies for categorical data to provide a ratio of the probability of the event occurring in the exposed group versus a non-exposed group.
- Pearson’s r (Correlation Coefficient) Pearson’s r measures the strength and direction of the linear relationship between two variables. Its absolute value can be interpreted as an effect size; the larger the absolute value of r, the stronger the relationship between the variables.
- R-Squared In the context of a regression analysis, the R-squared value represents the proportion of variance in the dependent variable which can be explained by the independent variables.
These types of effect sizes cater to different types of data and analyses, allowing researchers to quantify and communicate the practical significance of a wide range of findings.
Calculating Effect Size
- Cohen’s d This is calculated by subtracting the mean of one group from the mean of another group and dividing the result by the pooled standard deviation. It is often used in t-tests.
Cohen's d = (Mean group 2 - Mean group 1) / SDpooled
The pooled standard deviation is the square root of the average of the squared standard deviations. - Cohen’s f Cohen’s f is calculated by taking the square root of the ratio of the explained variance to the unexplained variance.
Cohen's f = sqrt[(SSeffect - SSerror) / SSerror]
- Eta Squared (η²) Eta squared is the proportion of total variation attributable to the factor, i.e., sum of squares between groups / total sum of squares.
η² = SSbetween / SStotal
- Cohen’s h This is calculated by taking the absolute difference between two proportions or rates.
Cohen’s h = 2 * arcsin(sqrt(p1)) - 2 * arcsin(sqrt(p2))
- Odds Ratio (OR) The odds ratio is calculated by dividing the odds of an event in the treatment group by the odds of an event in the control group.
OR = (odds in treatment group) / (odds in control group)
- Relative Risk (RR) The relative risk is the ratio of the probability of an event occurring in an exposed group to the probability of the event occurring in a comparison, non-exposed group.
RR = (Incidence in exposed group) / (Incidence in non-exposed group)
- Pearson’s r (Correlation Coefficient) Pearson’s correlation coefficient is calculated using this formula:
r = ∑(xi - X)(yi - Y) / sqrt[(∑(xi - X)²(∑(yi - Y)²)]
where: – xi and yi are the individual sample points indexed with i – X and Y are the means of the x and y variables - R-Squared R-squared is the square of the Pearson correlation coefficient.
It’s important to note that these are just the basic calculations for the different types of effect sizes. The actual calculations can get more complex depending on the structure of the data and the specific requirements of the analysis.
Interpreting Effect Size
Interpreting effect size is a key part of any statistical analysis. Here’s a general guide on how to interpret them:
- Cohen’s d and f According to Cohen’s original guidelines, a small effect size is 0.2, a medium size is 0.5, and a large size is 0.8 for Cohen’s d, and 0.1, 0.25, and 0.4 for Cohen’s f, respectively. However, these are just rules of thumb, and the context of the research should also be considered.
- Eta Squared (η²) Eta Squared is interpreted as the proportion of total variance in the dependent variable that can be explained by the independent variable. For instance, an Eta Squared of 0.06 would mean that 6% of the total variance can be explained by the independent variable.
- Cohen’s h There are no fixed guidelines for interpreting Cohen’s h, but a value of 0.2 is often considered small, 0.5 medium, and 0.8 large.
- Odds Ratio (OR) An OR of 1 implies that the event is equally likely in both groups. An OR greater than 1 implies that the event is more likely in the first group. An OR less than 1 implies that the event is less likely in the first group.
- Relative Risk (RR) A RR of 1 means there’s no difference in risk between the two groups. A RR greater than 1 means the event is more likely to occur in the first group, and a RR less than 1 means the event is less likely.
- Pearson’s r A correlation coefficient (r) of 0 implies no correlation. A coefficient of 1 implies a perfect positive correlation, while a coefficient of -1 implies a perfect negative correlation.
- R-squared R-squared represents the proportion of the variance for a dependent variable that’s explained by an independent variable. So, an R-squared of 0.20 means that 20% of the variation can be explained by the regression model.
Remember, while effect sizes can give us a measure of the strength of a relationship or the magnitude of a difference, they cannot provide information about the statistical significance of a result. To determine whether the effect is statistically significant, hypothesis testing is typically used in conjunction with its calculation.
Applications of Effect Size
Effect size has a variety of applications across multiple fields and research areas. Some notable uses include:
- Inferential Statistics Effect sizes are crucial in inferential statistics, where they help quantify the size of the difference between groups and provide a more reliable measure of effect magnitude compared to p-values.
- Meta-Analysis Effect sizes are used in meta-analyses to combine results from multiple studies, allowing for the comparison of effects across different datasets and providing a measure of the average effect and its variability.
- Power Analysis Effect size is essential in power analysis, helping determine the sample size needed to detect an effect of a given size with a given level of confidence.
- Social Sciences and Education Effect sizes are utilized in fields like psychology, education, and social sciences to assess the impact of interventions or treatments, such as evaluating the effectiveness of a new teaching method on student performance.
- Medical and Clinical Research Effect size is employed in medical research to estimate the efficacy of drugs or treatment methods and is used in clinical significance testing to evaluate the practical importance of treatment effects.
- Business and Economics In the business and economics fields, effect size can quantify the impact of business strategies or economic policies, allowing for comparisons between different interventions.
Understanding effect size is crucial in research and data analysis as it moves beyond binary conclusions and provides insights into the magnitude of effects. This knowledge drives decision-making and strategy development across a range of disciplines.
Limitations of Effect Size
While the concept of effect size is an invaluable tool in many aspects of research and data analysis, there are several important limitations to be aware of:
- Dependent on Context Effect sizes can vary in interpretation depending on the field of study, research question, and context in which they are used.
- Arbitrary Thresholds Interpretation of effect sizes is often guided by arbitrary thresholds, which can vary across fields and may not universally apply.
- Doesn’t Indicate Statistical Significance Effect size alone does not determine statistical significance; it is possible to have a large effect that is not statistically significant or a small effect that is statistically significant.
- Assumptions and Biases Effect sizes are influenced by assumptions made during calculation and can be affected by biases in the data or study design, such as outliers or skewed distributions.
- Not a Measure of Practical Significance Effect size measures the magnitude of an effect but does not directly reflect practical significance, which may depend on factors beyond the statistical magnitude.
Despite these limitations, effect sizes remain a valuable tool in research, providing a means to quantify and compare the magnitude of effects across studies. It is important to interpret it in the context of specific research questions and to consider statistical significance and practical implications alongside its various measurements.
Examples of Effect Size
Effect size is a universal concept used across many fields of study. Here are a few examples:
- Psychology In a study measuring the effectiveness of a therapy method on reducing anxiety levels, the calculated effect size (e.g., Cohen’s d) might be 0.8, indicating a large size according to Cohen’s guidelines.
- Education A researcher studying the impact of a new teaching strategy on student test scores could calculate the effect size by comparing the average scores of students who received the new strategy versus those who didn’t. A large size would indicate a substantial improvement in test scores.
- Medicine In a clinical trial evaluating a new drug, effect size can quantify the difference in health outcomes between a treatment group and a control group. The effect size might be reported as a risk ratio, odds ratio, or standardized mean difference, providing insights into the practical significance of the drug’s effect.
- Economics An economic study examining the impact of a policy change on employment rates might calculate an effect size to assess the magnitude of the policy’s impact. A high effect size would indicate a significant effect on employment rates.
FAQs
Effect size is a measure that quantifies the magnitude or strength of the relationship between variables in a statistical analysis.
Effect size helps to determine the practical significance of research findings and allows for meaningful comparisons across studies.
The calculation of effect size depends on the type of analysis being conducted. Commonly used measures include Cohen’s d, Pearson’s r, and odds ratios.
A large effect size indicates a substantial and meaningful relationship between variables, suggesting a strong impact or association.
About Paul
Paul Boyce is an economics editor with over 10 years experience in the industry. Currently working as a consultant within the financial services sector, Paul is the CEO and chief editor of BoyceWire. He has written publications for FEE, the Mises Institute, and many others.
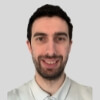
Further Reading
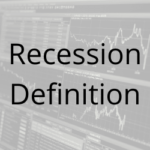
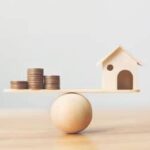
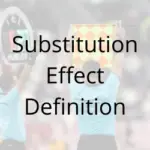