Anova: Definition, Types & Examples
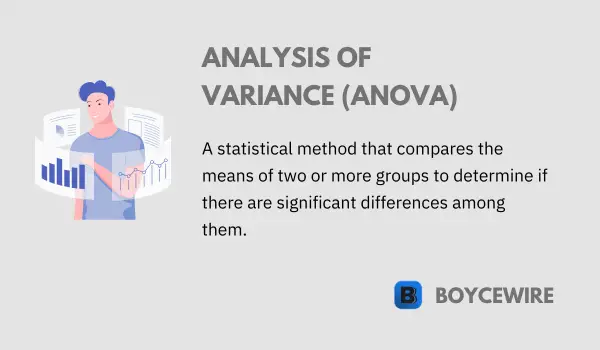
What is Anova?
Analysis of Variance, commonly known as ANOVA, is a statistical method used to analyze differences among group means. It was developed by renowned statistician Sir Ronald A. Fisher in the early 20th century, and it has since become an essential tool for researchers in various fields, from psychology to agriculture, business, and more.
ANOVA provides a statistical test of whether two or more population means are equal, and therefore generalizes the t-test beyond two means. Instead of looking at the difference between two means, it examines the ratio of variability between two conditions to the variability within each condition. It is typically used in experiments or observational studies with three or more groups, or in a setting where multiple variables might affect a single outcome.
In essence, ANOVA tests the impact of one or more factors by comparing the means of different samples, allowing researchers to study patterned differences between means and explore interactions between variables. This introductory overview will guide you through the foundational concepts of ANOVA, its applications, and its significance in statistical analysis.
Key Points
- ANOVA is a statistical method used to compare the means of two or more groups or treatments to determine if there are significant differences.
- It helps in understanding if the observed differences among groups are due to actual treatment effects or random variation.
- ANOVA calculates the F-statistic, which compares the variability between groups to the variability within groups.
Understanding ANOVA
Analysis of Variance, or ANOVA, is a statistical method used to compare the means of two or more groups to determine if they are significantly different from each other. It’s an extension of the t-test, which is designed for comparing only two groups.
It works by analyzing variance in the data – as the name suggests – to understand if the variation is due more to changes between groups or within the groups themselves. The null hypothesis in ANOVA is that all group means are the same, while the alternative hypothesis is that at least one group mean is different.
Here is a simplified way to understand how it works:
- Within-group Variance: This refers to the variability of the observations within each group. For example, if we are comparing test scores from three different classrooms, the within-group variance is the variance in test scores within each individual classroom. If this is high, it may affect the overall results of the calculation.
- Between-group Variance: This refers to the variability between the group averages. For example, the difference in average test scores between the three classrooms. The larger the between-group variance, the more likely it is that there are significant differences between the groups.
The ANOVA test is based on two estimates of the population variance (σ²). It tests the hypothesis that the group means are equal (the null hypothesis) by comparing the ‘between groups’ variance estimate with the ‘within groups’ variance estimate.
When the variance of the means of the groups (the between-group variance) is more than what you would expect by chance (the within-group variance), the test statistic will be large, and you reject the null hypothesis of equal means.
ANOVA produces an F-statistic: if the null hypothesis is true, this statistic follows an F-distribution. The F-statistic is the ratio of the mean squared error of the model and the mean squared error of the residuals. The larger the F-statistic, the less likely it is that the differences in group means are due to random chance.
However, while it will tell you if there is a significant difference between groups, it will not tell you which groups are different. To determine that, you would need to use a technique such as post hoc testing or planned contrasts.
Finally, it’s crucial to understand that ANOVA makes several assumptions, including that the data is normally distributed, that the variances of the populations are equal (homoscedasticity), and that the observations are independent. If these assumptions are violated, the results may not be valid.
Types of ANOVA
ANOVA can be broken down into two primary types, each suited to a specific kind of research question:
One-Way:
Also known as single-factor ANOVA, this type of analysis is used when there is only one independent variable. This variable typically has more than two levels or groups. The one-way ANOVA is used to determine whether there are any statistically significant differences between the means of three or more independent groups.
Two-Way:
This type, also known as factorial ANOVA, involves two independent variables. It is used to understand if there is an interaction between the two independent variables on the dependent variable. In other words, it allows the analysis of the effect of one variable on the outcome while considering the effect of another variable. It answers questions like, “Is there a difference in means due to one factor, the other factor, or both?”.
There are also other advanced forms, such as:
- Repeated Measures ANOVA: Used when the same subjects are used for each treatment (e.g., in a longitudinal study).
- Multivariate Analysis of Variance (MANOVA): Extends the capabilities of ANOVA to analyze multiple dependent variables simultaneously.
- Analysis of Covariance (ANCOVA): It combines ANOVA and regression. ANCOVA tests whether certain factors have an effect on the outcome variable after removing the variance for which quantitative predictors (covariates) account.
The choice of which type to use depends on the number of independent variables, the number of observations, and whether the observations are related or independent of each other.
How to Conduct an ANOVA Test
Conducting an ANOVA test requires several key steps. Here’s a general outline of the process:
-
Define Null and Alternative Hypotheses:
The null hypothesis (H0) in an analysis of variation test is that all group means are the same. The alternative hypothesis (H1) is that at least one group mean is different.
-
Organize Your Data:
Your data should be organized into a structured format. Typically, you’ll have different groups (like different treatments or categories) in columns and individual observations in rows.
-
Check Assumptions:
Before running ANOVA, it’s important to check if the assumptions of the test are met. These include:
- Normality: The data in each group should be approximately normally distributed. This can be checked with plots or normality tests.
- Homogeneity of variance: The variance within each group should be approximately equal. This can be tested using Levene’s test or Bartlett’s test.
- Independence: The observations should be independent of each other.
-
Calculate ANOVA:
Depending on your data and the software you’re using, the way you calculate ANOVA may vary. However, most statistical software packages (like SPSS, R, or Python’s scipy library) can perform such tests.
-
Check the P-value:
After you’ve run the test, you’ll want to check the associated p-value. If this value is less than your significance level (commonly 0.05), you reject the null hypothesis and conclude that there is a significant difference between at least two of the group means.
-
Perform Post-Hoc Tests if Necessary:
If you have more than two groups and your ANOVA test is significant, you may want to perform post-hoc tests to determine which specific groups differ from each other.
-
Report Your Results:
After analyzing the data, the final step is to present your results. This will usually involve reporting the F statistic, degrees of freedom, and p-value from the ANOVA, along with any significant results from post-hoc tests.
Remember, conducting an ANOVA requires a careful understanding of your data and the assumptions of the test. Be sure to explore your data thoroughly before conducting the analysis and interpret the results with caution.
Examples of ANOVA in Real-World Research
ANOVA (Analysis of Variance) is an essential tool used in a multitude of fields and disciplines. Its application allows researchers to compare the means of different groups and better understand the data at hand. Here are a few examples of how ANOVA is used in real-world research:
1. Medicine and Health Sciences
It can be used to compare the effectiveness of different treatments or interventions. For instance, researchers might compare the mean blood pressure levels of patients undergoing three different treatments for hypertension to determine which is the most effective.
2. Psychology
In psychology, it is often used in experimental studies. A researcher could use ANOVA to investigate whether different types of therapy have different effects on levels of depression in patients.
3. Agriculture
In agricultural research, ANOVA can be used to compare crop yields under different treatment conditions, such as varying amounts of fertilizer, different irrigation methods, or different types of seed.
4. Marketing
A marketer might use it to compare the effectiveness of different advertising strategies on different demographic groups. The mean response (such as purchase intent or brand recognition) could be compared across different advertising approaches.
5. Education
In education research, it might be used to compare the mean test scores of students taught with different teaching methods, or in different types of classroom environments.
FAQs
ANOVA is a statistical technique used to analyze the differences between the means of two or more groups or treatments to determine if there are significant variations among them.
The purpose of ANOVA is to determine if there is a statistically significant difference in means among groups, helping researchers understand the impact of different variables or treatments on the observed outcomes.
ANOVA should be used when there are three or more groups or treatments being compared, and researchers want to determine if there are significant differences in their means.
Some assumptions include independence of observations, normal distribution of residuals, homogeneity of variances, and equal group sizes.
About Paul
Paul Boyce is an economics editor with over 10 years experience in the industry. Currently working as a consultant within the financial services sector, Paul is the CEO and chief editor of BoyceWire. He has written publications for FEE, the Mises Institute, and many others.
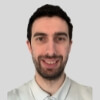
Further Reading
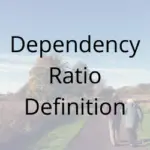
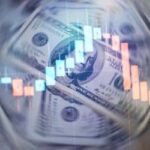
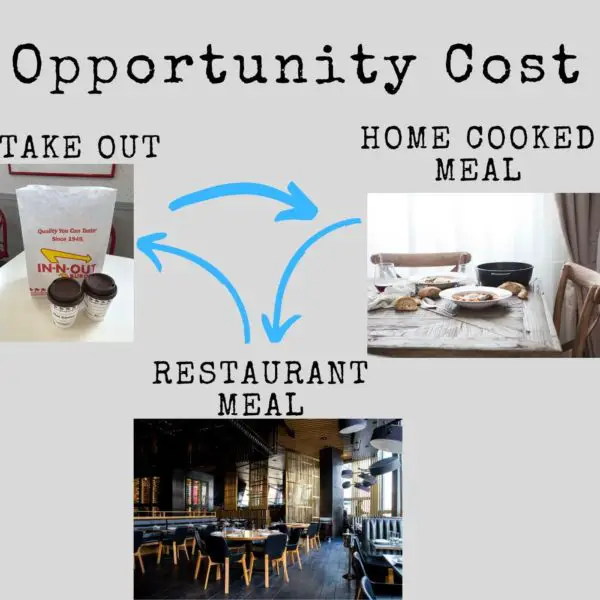